AFK, or Agency for Finance in Kosovo, is one of the largest microfinance institution in the country that offers individual loan methodologies with the goal of improving living conditions in Kosovo by providing sustainable financial services to micro and small businesses.
Aspekt has implemented an advanced and analytical predictive model, which is boosting working process efficiency and allowing flexibility while making better, faster, and more accurate decisions. The project was supported by Incofin as a respectful, accessible and practical investor. They have recognized the importance and the need of this project for AFK as a crucial step in getting deep into machine learning and modeling the AFK’s data to solve different business problems and cases.
- Exploratory Data Analysis
- Statistical Modeling
- Predictive Analytics
Aspekt gave its expertise in business analysis and, as a result, determined what type of model was required to lower the risk portfolio and reduce default and delinquent loans. When we talk about defaulted and delinquent loans, we’re referring to loans with late payments that have a negative impact on the client’s credit record. We proceeded by gaining a thorough understanding of the business and AFK’s strategy, as well as summarizing their data and establishing the analytics environment.
Platform that understands the Data
- Exploratory Data Analysis
- Descriptive & Diagnostic Statistic
- Data Visualization & Storytelling
- Data Transformation and Modeling
- Exploring Relationships and
Investigate Patterns - Recognizing Correlations, Define
Variables of Interest - Predictive Analytics & Anticipation
of Certain Results - Creation of More Accurate and Faster Decisions
- Deep Data Insights and Improved Automation
- Monitor KPIs and Trends as They Change in Real-time
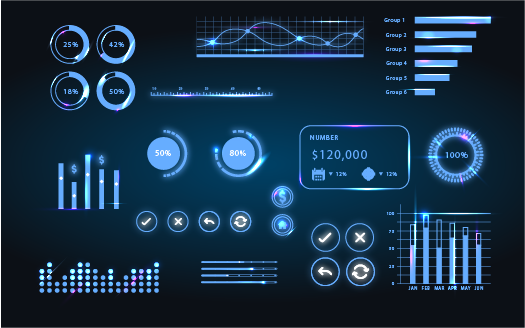
Predictive Analytic Model for
Delinquency and Loan Default Risk
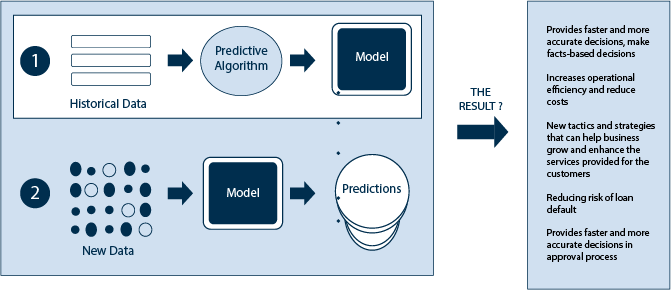
Machine Learning Models
Capable of quickly integrating the most recent data when conditions in the borrower’s life change and forecasting delinquency even before it arises. This knowledge can assist MFIs in taking prompt action to reduce delinquency, safeguard cash flow liquidity, and segment borrowers based on risk indicators. Furthermore, Machine Learning-powered solutions may provide real-time resource allocation recommendations or offer alternative payment dates.
EDA (Exploratory Data Analysis)
EDA is a crucial step to take before diving into machine learning or statistical modeling. It provides the context needed to develop an appropriate model for the problem and to correctly interpret its results. EDA was performed to make certain that the produced results are valid, correctly interpreted, and applicable to the desired business contexts.
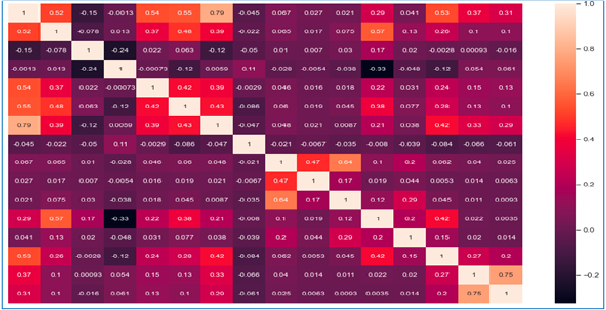
Through EDA we discovered hidden trends, tested a hypothesis and checked assumptions to obtain the reasons for late loan repayment. By analyzing certain characteristics such as social characteristics of clients, clients’ balances and cashflows, products provided by AFK, AFK branches etc., we have discovered relationships and patterns that helped us decide which model is best for our Client.
The Data is a great asset that can-do wonders for improving the businesses
With the help of different data visualization methods and techniques, we summarized particular characteristics of datasets and represented it in a simple, more acceptable way for a human eye.
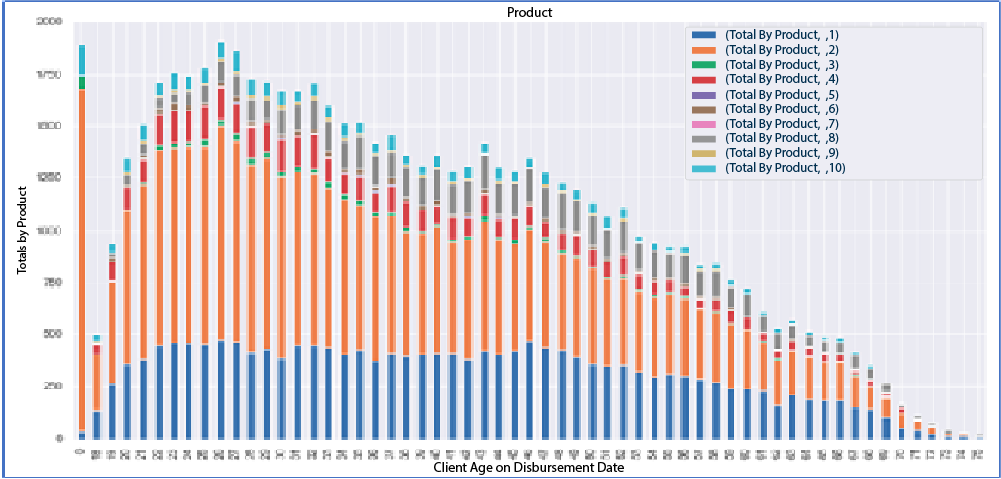
Supervised Machine Learning
Model has been developed using Supervised Machine Learning. Several Machine learning algorithms were tested and compared, examining current and historical data and projecting what we discover onto a final model creation to foresee expected events. The final model is based on the Random Forest algorithm as it provides us the best results and performance for the defined business problem and used historical data. The forest it builds is an ensemble and combine multiple models of decision trees, a collection of uncorrelated decision trees which are then merged together to reduce variance and create more accurate predictions.
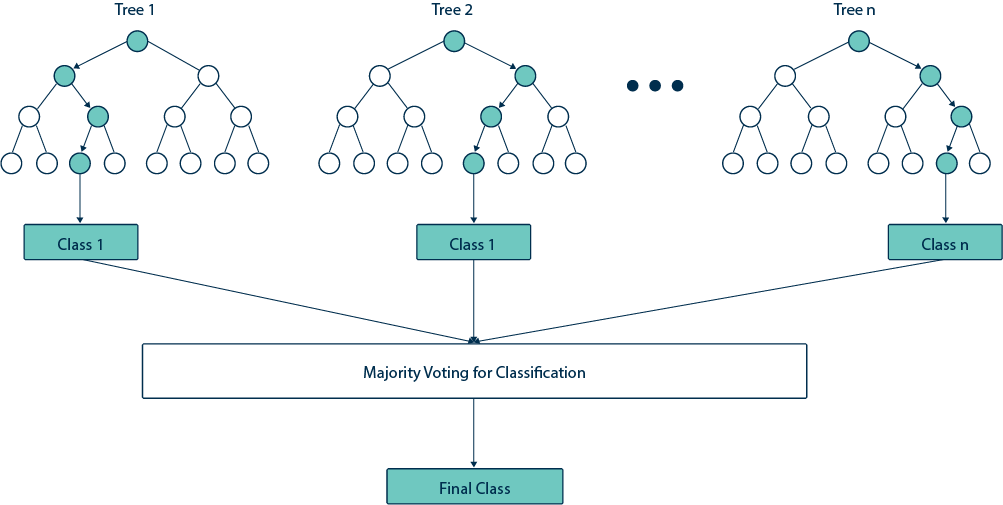
Leading questions used for delivering the Model assumptions and making predictions
- What is the overall success, failure trend for a particular period related to loan sizes and terms?
- Which particular period, months, year, have the greatest number of delinquency/defaults?
- Which regions, branches, offices are most responsible for delinquent or default?
- Which are the top branches, offices with the most delinquency/defaulters?
- Which are the top products having the least/most contribution towards the delinquency/default?
- Which customers have the greatest number of delinquency/defaults?
- What are the common reasons causing defaults?
Model Approach Benefits
Our solution provides AFK with an engine within the core system, fully automated operations, real-time feedback, numerous estimations based on past and the most recent data, detailed statistics and 360-degree view of the clients as well as data summarization and visualization and predictive analysis.
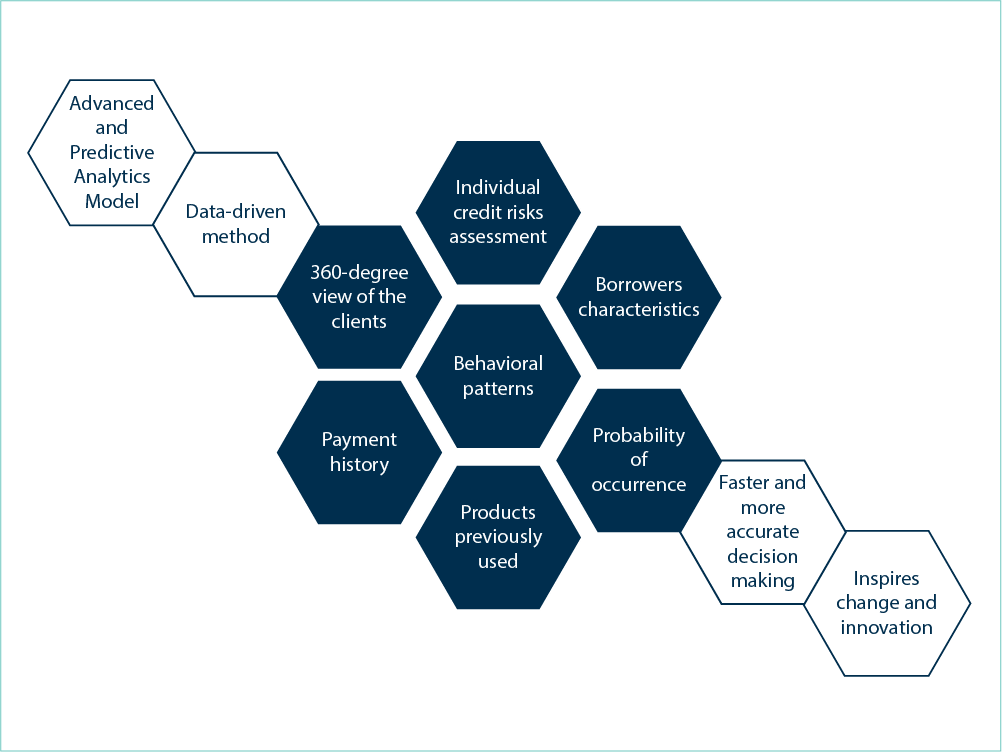